Predicting Tracking Performance of AR-Image Markers
Yan Walesch
Master's Thesis, August 2023
Supervisors: Börge Scheel, Daniel Vera Nieto, Henry Raymond, Dr. Fabio Zünd, Prof. Dr. Bob Sumner
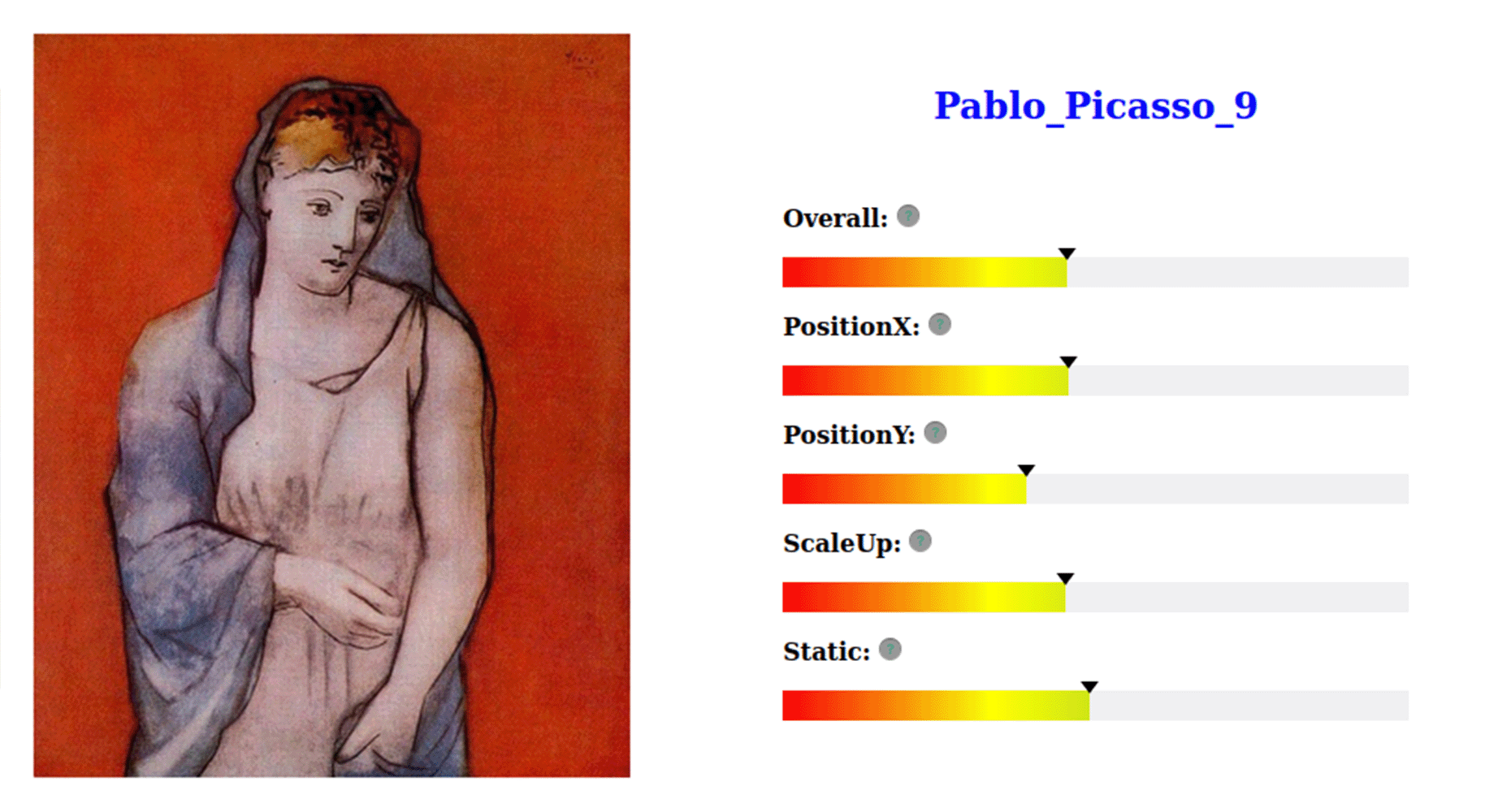
Abstract
Marker-based tracking is one of the most used methods for Augmented Reality (AR) applications. The concept involves the use of images or printed patterns called markers that are placed as reference points in the physical world. The design of these markers is crucial to the effectiveness of the tracking algorithm and plays a major role in the performance of many AR applications. However, identifying a marker that can be conveniently tracked and simultaneously contributes to the aesthetic of the experiment represents a challenging endeavour. Moreover, specific use cases do not permit the selection of marker design, therefore determining whether predefined images would suffice is an essential preliminary requirement for any given application. In particular, we focus on the use case of museum paintings where there’s no possibility of marker design as the paintings cannot be modified.
This research explores the potential of utilizing Machine Learning (ML) methods to ascertain the performance of any image as an AR marker. To achieve this goal, we introduce a novel data set composed of famous paintings coupled with measured tracking times in four different scenarios close to real world use cases. Additionally, we provide improvements to the measuring tools employed in prior work, concerning data management and storage. Subsequently, we carry out a comprehensive empirical study of the performance of different Deep Learning (DL) and ML models. Finally, we showcase the performance of the models by providing a user-friendly web application designed to predict the performances of uploaded image data thereby improving the marker selection process.